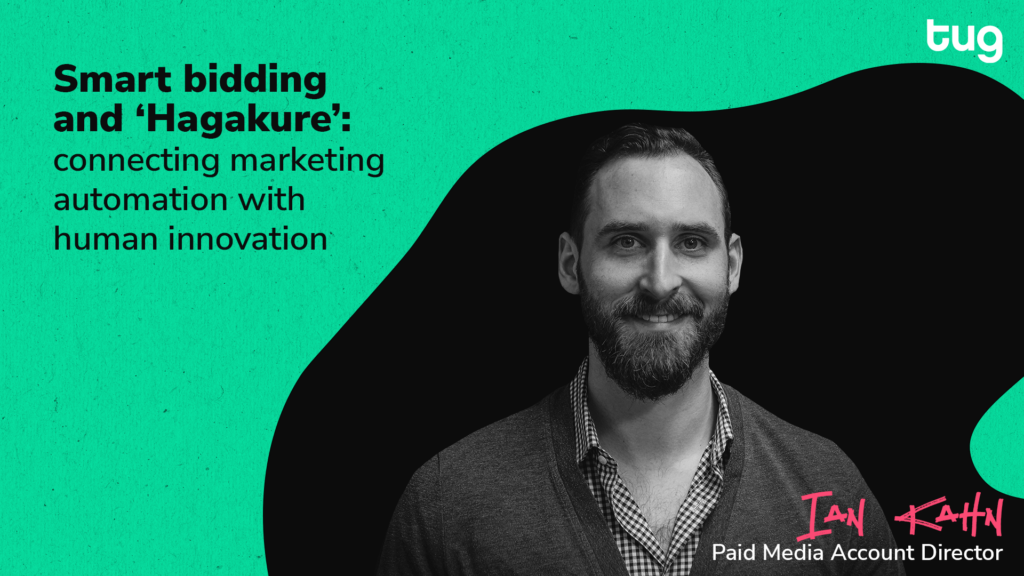
Embracing the Paradigm Shift: Connecting Marketing Automation with Human Innovation
Machine Learning technology (or ML as it’s also known) looks set to revolutionise paid ad campaigns, leading to a seismic paradigm shift which replaces manual bidding in favour of smart bidding.
Manual bidding involves PPC experts setting their maximum cost-per-click (CPC) to optimise campaigns. In comparison, smart bidding allows ML tech to determine the ideal bid amount for individual searches in real-time, based on data, helping to increase conversions while saving time and money. Emerging in 2016, smart bidding seems to be a significant focus for Google as they continually develop their ad systems and move beyond traditional keyword targeting. Therefore, learning how to structure ad accounts effectively is crucial for success with smart bidding.
To make the most efficient use of ML, we can look to a new machine-learning account structure known as “Hagakure.” Originating from Samurai literature, the phrase roughly translates to “hidden behind the leaves” and represents a practical and spiritual guide for a Samurai warrior.
In Paid Search Marketing, the strategy involves simplification of a Google Ads account structure to maximise the potential of ML. The aim is fewer campaigns and ad groups with less segmentation. Instead, you want to create sizeable ad groups that bunch a large amount of data. This helps Google’s algorithms identify and maximise the potential of ads more quickly and effectively. Combining this structure with smart bidding, the “Hagakure” strategy shifts the focus away from granular account management previously seen in manual bidding, and prioritises data.
When applying machine learning technology and a “Hagakure” account structure, marketers must first identify their goals for a ML-optimised account and be able to measure them accurately. Think about the areas where you want to see improvement, and where automation can have a significant impact. Below are some KPIs for a well-optimised, ML-friendly account that you might incorporate into your strategy:
Data dictates how well a machine learning system performs. PPC experts must collect, manage, and curate the right data for the campaign and brand in question. Not doing so can result in inaccurate learning – and the machine will fail to achieve its purpose. Human-technology collaboration is essential to the success of content marketing automation. It’s not just a case of setting up the system and leaving it to run, however. The results of the BCG Digital Marketing Maturity Study, 2019 (below), demonstrate the performance differences between tech without human input – and tech with human input.
Making advertising campaigns work harder is part of what we do at Tug Agency. Our Paid Media Account Director Ian Kahn presented the “Hagakure” method to attendees of DMEXCO 2022. He used our experience with Pabio – a subscription-based furniture and interior design platform – to evaluate the effectiveness of this smart bidding account structure.
As a response to a drop in customer numbers, Tug was tasked with generating new qualified leads and subscriptions. To achieve this, we sought to improve the metrics of CPL (cost per lead), CAC (cost per acquired customer) and TCV (total contract value). Through our collaboration with Pabio, and the application of the “Hagakure” methodology, we implemented key strategic changes including:
- Reduced ad campaigns – from 120 to 16. Achieved by removing all match type and device segmentation while consolidating ad groups and campaigns for ML-friendly bidding.
- Better use of available data to measure qualified leads, floor plan uploads, and paid subscriptions, with upgrades to conversion tracking and bid strategy goals.
- Refined the onboarding process by adding a budget selector, enabling us to measure dynamic lead value for each individual lead.
- A switch to 90% broad match KWs and 10% exact match for brand terms.
- Added more generic furniture terms to increase reach and enable sustainable growth through machine learning.
The results are not only impressive, but they also showcase the value of ML and human collaboration. After the first three months we saw:
- 76% improvement in cost per lead (CPL) by consolidating the machine learning campaign structure and data-driven targeting.
- 47% reduction in the cost per click (CPC) across paid media channels.
- 50% increase in average new contract value generated by paid media activity.
- 30% of new qualified leads were acquired from generic search terms.
With an increasingly automated industry, evident through the success of Tug + Pabio collaboration, marketers must look to the future. With this in mind, what key aspects will impact the application of ML in paid activity?
“As machine learning in advertising continues to advance, advertisers must be willing to re-evaluate best practices. They should shift focus towards ensuring the right data is fed back into advertising campaigns, rather than focusing on granular keyword optimisations, ad group segmentations, and manual bid adjustment. This creates a feedback loop which accelerates efficient growth and exceeds the optimisation possibilities of manual strategies.
Additionally, advertisers must develop new ways of working and acquire new skill sets. With this, an advertiser can help clients and stakeholders develop their own understanding of the data’s importance and how to best establish a privacy-safe end-to-end tracking solution. Being able to track each stage of the customer journey from first to last touchpoint and feeding that data back to Google is becoming the most important driver of performance. As third-party cookies slowly become a thing of the past, ensuring that first-party data is collected accurately and used securely will become paramount to future online marketing success.“ Ian Kahn, Paid Media Account Director